[ad_1]
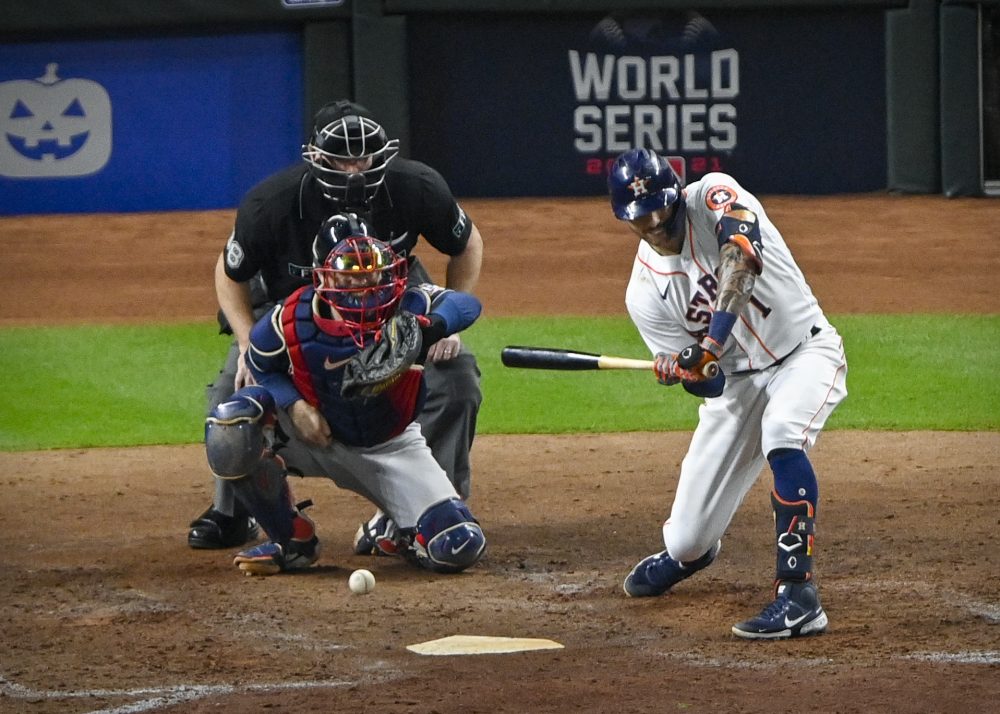
Picture credit score: © Jerome Miron-USA TODAY Sports activities
This idea was initially introduced at a SABR convention in March 2022 and has been tailored for publication at Baseball Prospectus. Half 2 will comply with tomorrow.
Plate self-discipline is a side of baseball that has continued to be investigated as an essential a part of hitting efficiency. On this piece, we describe a brand new metric Anticipated Extra runs Gained by Trying/swinging Estimate (EAGLE) developed to precisely quantify how good a participant is at making choices on the plate. The plate self-discipline revolution began when the conclusion that taking walks can contribute tremendously to run scoring helped launch the Moneyball period. In 2002, the Oakland Athletics found this phenomenon and found that there have been a major variety of baseball gamers who have been undervalued due to some attributes like age, capability to throw, or lack of velocity. In the end, these traits didn’t cowl the whole lot of the explanations these gamers have been undervalued. Baseball since its inception has at all times valued gamers who may hit the ball greater than gamers who couldn’t and it was just about that easy. Now, we all know higher.
Baseball analytics have superior to the purpose the place we worth stroll charges and chase charges extremely and take a look at in-zone and out-of-zone swing and get in touch with charges as nicely to find out how good a participant is at “plate self-discipline.” However, plate self-discipline is greater than merely drawing walks and avoiding strikeouts. Fairly, it’s about each determination a hitter makes to swing or to not swing. We sought to quantify that call on a extra in depth degree than simply whether or not or not the batter makes it to ball 4 or swings at a pitch exterior the strike zone.
Not each pitch within the strike zone is essentially pitch for the batter to swing at. Not all strikes are created equal. A fastball on the surface nook is way more troublesome to hit than a dangling curve in the midst of the zone; a hitter is extra more likely to get consequence by swinging on the hanging curve and taking the fastball for a strike, particularly in a positive depend. Moreover, a fastball on the surface nook may be referred to as a ball. All of this needs to be taken under consideration when evaluating the choice of the hitter to swing the bat or to let it drop.
In our strategy to evaluating this determination, we broke down the attainable outcomes of every determination the hitter may make into the tree under.
The outcomes of the hitter taking the pitch are comparatively simple, with both a strike or a ball occurring based mostly on the umpire’s determination, however there are numerous potential outcomes when the hitter swings the bat. Due to this, we narrowed it all the way down to the seven outcomes pictured: Miss, Foul, Out, Single, Double, Triple, or Homerun.
From right here we would have liked to find out what the bottom metric needs to be, in different phrases, what ought to we be quantifying? Instantly, runs is a really engaging type of items for this metric. Fortunately, this may be completed with delta run expectancy, devised by Tom Tango. This metric is calculated by subtracting the preliminary run expectancy from the ultimate run expectancy or, the run expectancy after the pitch. This makes use of a run expectancy matrix just like the one under, however together with depend as nicely as a result of we’re coping with a pitch by pitch strategy.
Now that we’ve got this deliberate out, we have been in a position to begin the method of accumulating knowledge and constructing the fashions essential to create EAGLE. We utilized the baseballr bundle to scrape baseball savant knowledge from the 2019 and 2020 baseball seasons. From right here, we merged the delta run expectations for each attainable consequence to every particular pitch in order that we now have the muse laid out and have the runs added (or subtracted) for the entire outcomes that we’ve got outlined.
Now that we’ve got the run expectations of every attainable consequence, we have to know the possibilities of every of those outcomes occurring and should perceive the impact on run expectancy for of the 9 outcomes we specified.
To calculate the run expectancy for every determination, we’d like the likelihood every consequence will happen, and the anticipated runs if it does. We predict the likelihood a pitch will probably be referred to as a strike or a ball if the hitter doesn’t swing, and calculate the anticipated runs for every of these outcomes. Combining these offers us the anticipated runs within the inning if the hitter takes the pitch. We additionally have to estimate the likelihood of every of the seven outcomes if a hitter have been to swing at every pitch. We mix these estimates with the anticipated runs for every consequence to calculate the anticipated runs if the hitter have been to swing.
As a way to predict the likelihood of a strike, we used an XGBoost mannequin with a logistic goal to be able to seize the nonlinearity of umpires’ choices. Ultimately, the impartial variables for the mannequin have been x-location, z-location, and variables for the highest and backside of the strike zone. The mannequin has an AUC of 0.982, and the estimates of the strike zone for a league-average peak hitter are proven under:
To foretell consequence chances for the swing determination, we skilled an XGBoost classification mannequin. We utilized pitch data options like velocity, spin price, motion, location, and kind in addition to situational data like depend, outs, and pitcher/batter handedness. We additionally included descriptive statistics concerning the batter and pitcher listed under:
- Hitter
- PA, AVG, OBP, SLG
- EV, LA, xBA, xwOBA, wOBA, ISO
- Pitcher
- IP, ERA, WHIP, GB/FB, Ok,BB%
- Common Velocity, Common Spin Charge
The mannequin was tuned utilizing cross validation to find out the optimum meta-parameters for the mannequin. On this method we have been in a position to cut back the imply squared error and find yourself with the ensuing confusion matrix proven under of chances, the true outcomes being the columns and the typical predicted chances given within the rows. For instance, for every pitch which was truly hit for a double, we predicted a 16.0% likelihood of a swing and miss, a 41.2% likelihood of a foul ball, and a 4.2% likelihood of a double (amongst others). For missed pitches, we estimated a 40.98% likelihood of a miss, a 32.8% likelihood of a foul, and solely a 1.06% likelihood of a house run.
To mix the outputs right into a single complete metric, we multiply every consequence likelihood by the change in run expectancy for that consequence. We add the outcomes collectively and do that for each the take outcomes and the swing outcomes as within the formulation under.
We then find yourself with a run expectancy for every determination, for swing and for take. We choose the right determination because the change in run expectancy that’s the bigger of the 2 and the participant’s EAGLE for that pitch is the distinction between the choice taken and the opposite alternative. That is the anticipated Runs Gained metric outlined right here. Then EAGLE is simply the anticipated Runs Gained based mostly on the choice made.
Bringing this all collectively we will illustrate this within the determination diagram from earlier than as you see under:
Sadly, run expectancy isn’t as simple as this. When Mike Trout comes as much as the plate, you’d anticipate a special variety of runs to be scored than when Freddy Galvis does. To regulate for this, we regressed delta run expectancy on each the state of affairs and the hitter’s OPS. Then we adjusted the unique run expectations of the conditions by these corresponding predicted delta run expectations for the hitter. To indicate the connection between delta run expectancy and a hitter’s OPS, we created the visible under.
Now that we’ve got the EAGLE metric, we will examine its effectiveness and what units it aside from different plate self-discipline metrics in addition to apply it on the person pitch and mixture degree within the subsequent article.
To be continued…
Joshua Mould is a Junior at Villanova College finding out Laptop Science and Statistics and will probably be an Affiliate Quantitative Analyst this summer season with the Philadelphia Phillies
David Anderson is the Michele and Christopher Iannaccone ‘91 Assistant Professor of Analytics on the Villanova Faculty of Enterprise
Thanks for studying
It is a free article. When you loved it, think about subscribing to Baseball Prospectus. Subscriptions assist ongoing public baseball analysis and evaluation in an more and more proprietary surroundings.
[ad_2]
Source link